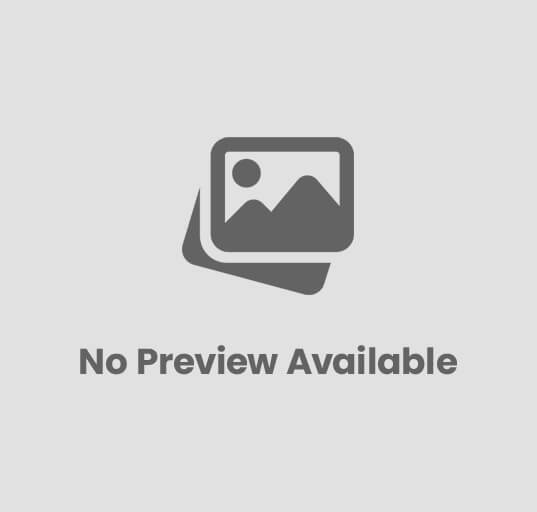
AI Model Audits Require a ‘Trust, but Verify’ Strategy to Improve Dependability
The quest for reliability in the growing field of AI models continues to be challenging, particularly in critical sectors such as healthcare and finance. To bolster confidence in AI, conducting thorough audits of these models is crucial for regulators, developers, and users alike, ensuring enhanced accountability and adherence to guidelines.
However, the reliability of AI model audits can sometimes be questionable because auditors must meticulously examine the stages of pre-processing, in-processing, and post-processing. By implementing a ‘trust, but verify’ strategy, the integrity of the audit process can be improved, fostering greater public confidence in AI technologies.
Challenges of Conventional AI Model Audit Systems
AI model audits play a pivotal role in elucidating how AI systems operate, their potential implications, and delivering evidence-based assessments for industry stakeholders.
Organizations utilize these audits to guide their decisions on AI models by conducting due diligence and evaluating the comparative advantages of various vendors’ offerings. Additionally, these reports confirm that developers have implemented necessary precautions throughout all phases and ensure compliance with relevant regulations.
Despite their usefulness, the reliability of AI model audits is often compromised due to procedural limitations and challenges related to human resources.
The checklist from the European Data Protection Board (EDPB) indicates that audits conducted by a “controller’s implementation of the accountability principle” and “oversight by a Supervisory Authority” may yield inconsistent results, leading to potential confusion among enforcing bodies.
While the EDPB’s checklist addresses implementation methods, data verification, and subject impact through algorithmic audits, it acknowledges a significant gap: the audits do not assess whether a system should exist at all.
Moreover, audit teams must possess current knowledge in data science and machine learning. They also require access to comprehensive datasets for training, testing, and deployment, resulting in intricate workflows and dependencies.
A single knowledge shortfall or mistake within the auditing team can trigger a series of failures, potentially invalidating the entire audit outcome. As AI models evolve in complexity, auditors face growing demands to validate and verify reports before aggregating them for conformity checks and corrective actions.
The rapid advancement of the AI sector has outstripped the auditors’ ability to perform thorough analyses and evaluate AI models effectively. Consequently, this gap in auditing techniques and skill sets exacerbates the trust deficit surrounding AI model audits.
An auditor’s fundamental responsibility is to promote transparency by assessing risks, governance, and the core processes of AI models. If auditors lack the necessary expertise and resources to evaluate AI and its implementation, trust among users diminishes.
A report by Deloitte outlines three pivotal lines of defense in AI governance. The first line assigns primary responsibility for risk management to model owners and executives. The second line comprises policy experts who provide essential oversight for risk management.
The third, and arguably most crucial, line of defense encompasses auditors who analyze the effectiveness of the previous two lines and report their findings to the Board of Directors, summarizing insights on best practices and regulatory compliance for AI models.
To bolster the reliability of AI model audits, a ‘trust but verify’ mindset should be embraced by both the personnel and the technology involved during audit processes.
Implementing a ‘Trust, But Verify’ Framework for AI Model Audits
The phrase ‘trust, but verify’ gained prominence through U.S. President Ronald Reagan during discussions on nuclear arms treaties with the Soviet Union. Reagan emphasized the importance of “comprehensive verification processes that allow both parties to monitor adherence,” a principle that can help restore credibility in AI model audits.
Incorporating a ‘trust but verify’ framework mandates ongoing assessment and validation of AI model audit results before accepting them as accurate. Essentially, this establishes that the mere completion of an audit does not equate to inherent correctness.
Consequently, despite robust verification protocols and validation of all key elements, an AI audit can never be deemed completely dependable. Researchers Phil Laplante and Rick Kuhn describe this continuous verification approach within AI system architecture.
The critical need for ongoing assessment and constant assurance in AI is pivotal for the auditing of models. For instance, AI systems often necessitate re-evaluation and re-auditing as mission parameters or contexts evolve over time.
A ‘trust but verify’ audit methodology can facilitate the detection of model performance issues through advanced fault detection strategies. By implementing continuous monitoring, audit teams can deploy testing and mitigation methods that enhance the rigor of algorithms and oversight systems.
Laplante and Kuhn assert that “continuous supervision of the AI framework is a vital component of the post-deployment assurance model.” This level of oversight can be achieved through automated AI audits, integrating routine self-diagnostic processes within the system.
Since internal diagnostics can be met with skepticism, a hybrid approach that incorporates both human oversight and machine analysis can enhance the monitoring of AI. This dual system strengthens the auditing process by enabling thorough retrospective investigations and black box analyses for validating results against contextual references.
The primary role of an auditor is to ensure AI models remain within established trust boundaries. A ‘trust but verify’ methodology enables auditors to explicitly verify trustworthiness throughout every phase, addressing issues of reliability in AI model audits and reinstating confidence in these systems through meticulous evaluation and transparent practices.
Post Comment